Brian Malone, a writer for dynastyleaguefootball.com, has put together a great guest post today. You can follow him on Twitter at @BrianMaloneFF. Thanks to Brian for today’s article!
Testing the Tape
Projecting a player’s NFL potential from his college football performance is something like predicting a player’s potential as a tennis pro from his performance in the collegiate racquetball club. Sure, there’s correlation, but the variance in level of competition and style of play create ample noise. No wonder folks on Twitter spend hours debating hand size: at least it’s a standardized measure, and it’s not obviously worse than things like collegiate yards per carry.
The better approach is film study. Unfortunately, I’m not any good at it. Indeed, I don’t even know how to tell whether anyone else is any good at it. But that’s a problem we can attack. And the natural starting point is Matt Waldman’s Rookie Scouting Portfolio (RSP), which has the benefits of quantified observations, independent analysis, and a 10-year history.
The RSP includes a checklist of observed skills and abilities, including things like “Runs behind pads/Good pad level” and “Catches ball with proper hands technique.” The RSP assigns a weight to each and combines them into what I’ll call trait scores (i.e., “Power” and “Balance”) and an overall score.
Note the three steps to this process: (1) observing the skills and abilities; (2) assessing the importance of observed skills and abilities to each trait; and (3) assessing the importance of each trait to a player’s overall ability. The first step is off limits: we’ll take the observations as given. But the others are fair game: we have the tools to use Waldman’s observations, plus a little math, to build a traits-based model for predicting a prospect’s success.
Building and testing the model
The data
The RSP provide 10 years of observation data, from 2006 to 2015. It’s too early to judge the success of the 2015 rookie class, so we’re left with 154 eligible running back prospects – backs who were both drafted and included in the RSP. I built a model based on the 2006 to 2011 classes and tested it on the 2012 to 2014 classes.
Measuring success
To measure success, I used a player’s best fantasy points-per-game (using 0.5 PPR scoring) in any of his first three seasons, with a minimum of 10 games played. It’s not the ideal measure of running back production, but it’s what I had handy. If any player failed to play at least 10 games in any of his first 3 seasons, his results were not included.
This measure (1) illustrates a spectrum of success; (2) doesn’t unduly reward (or penalize) prospects who have more early-career opportunity; and (3) reflects fantasy football value. To avoid tainting the results, I chose this measure before I analyzed the observational data.
Building the model
The RSP includes nine traits for running backs: Power, Balance, Ball Handling, Speed, Vision, Elusiveness, Receiving, Blocking, and Durability. These traits, rather than their component skills and abilities, are the foundation of the model. [1]Some skills and abilities have been added or dropped since 2006. I charted only those that were present from 2006 through 2014. I assigned weights based on the 2014 RSP. For example, “Effective … Continue reading To build the model, I primarily used data snooping, which is why testing on a separate data set was necessary. I identified two types of running backs who had early career success.
- All-purpose backs – Speed > 11 (top 67%); Power > 16 (top 32%); Elusive > 11 (top 48%)
- Receiving backs – Speed = 12 (top 61%); Receiving ≥ 5 (top 32%); Blocking > 5 (top 31%)
Any back who fit both sets of criteria was listed as an all-purpose back. From 2006 to 2011, there were 14 all-purpose backs [2]Joseph Addai, Delone Carter, Jamaal Charles, Jonathan Dwyer, Matt Forte, Mark Ingram, Brian Leonard, Marshawn Lynch, Knowshon Moreno, Cedric Peerman, Adrian Peterson, Bilal Powell, Ray … Continue reading and 6 receiving backs; [3]Reggie Bush, Quinton Ganther, Felix Jones, Maurice Jones-Drew, Jerious Norwood, and Steve Slaton. the remaining 74 backs satisfied neither set of criteria. Despite what the percentiles suggest, the model’s trait scores are near the maximum possible. Indeed, the model has roughly a dozen knockout criteria: prospects who didn’t consistently demonstrate speed in the second level, for instance, couldn’t earn an 11 in the Speed trait.
I used a logarithmic best-fit line to calculate the expected maximum point-per-game season for each running back, based on NFL draft position. For example, Reggie Bush, who was drafted #2 overall, was expected to have a best season of 20.4 PPG. Rashad Jennings, drafted #250 overall, was expected to have a best season of 5.4 PPG. Compared to draft expectations, both types of qualifying backs outperformed their counterparts by almost 1.5 points per game, as this summary of averages shows:

Testing the model
I tested the model on running backs from the 2012 to 2014 draft class. I again used a logarithmic best-fit line to calculate each running back’s expected maximum PPG season based on draft position. I then identified running backs using the model’s criteria. The model identified six all-purpose backs (Le’Veon Bell, Marcus Lattimore, Trent Richardson, Bishop Sankey, Spencer Ware, and David Wilson) and two receiving backs (Devonta Freeman and Isaiah Pead) over this time frame. The remaining 48 backs satisfied neither set of criteria. Again, when compared to expectations based on draft position, both groups of qualifying backs outperformed their counterparts by about 1.5 PPG.
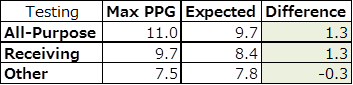
The testing sample was unexpectedly and unfortunately small. However, the results are promising, as both groups performed consistently with the model-building results.
If you’re curious, here’s a graph of every player’s maximum PPG season, plotted by NFL draft position. The logarithmic line represents the expected PPG at each draft position.
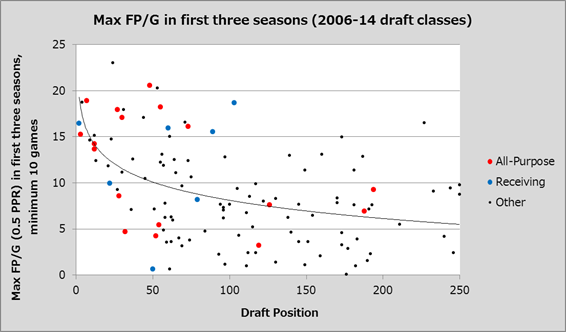
Unexamined data – the 2015 class
The model loved the 2015 class of running backs. Specifically, it identified eight all-purpose backs: Ameer Abdullah, David Cobb, David Johnson, Duke Johnson, Josh Robinson, Karlos Williams, T.J. Yeldon, and Todd Gurley. No receiving backs were identified. Ten backs fit neither set of criteria: Cameron Artis-Payne, Javorius Allen, Jay Ajayi, Jeremy Langford, Kenny Hilliard, Marcus Murphy, Melvin Gordon, Mike Davis, and Tevin Coleman. It is, of course, too early to measure the results of these predictions, but they appear promising at a glance.
Conclusions
As with any “beat the draft” hypothesis, this one risks the possibility that the NFL will quickly adjust (or has already adjusted). But that risk is small here, mainly because the magnitude of the proposed inefficiency is small and the evidence for it isn’t very strong. Until teams start cheating directly off the RSP, this inefficiency – if it exists – will be our secret.
Another concern is the evolution of Waldman’s evaluation process. Even where the RSP’s labels remain constant, Waldman’s assessments are almost certainly changing. In general, this should allow for stronger future models (as there’s no evidence Waldman’s getting worse over time). But that shouldn’t stop us from using the data we have now. And despite the changes in Waldman’s process, this model apparently predicted success in the 2012-14 (and maybe ’15) draft classes based on data from the 2006-11 classes.
For predicting NFL success, draft position is impossible to beat and difficult to supplement. There isn’t enough data here to draw confident conclusions, but there is enough to encourage my belief that film study is ripe for analysis.
References
↑1 | Some skills and abilities have been added or dropped since 2006. I charted only those that were present from 2006 through 2014. I assigned weights based on the 2014 RSP. For example, “Effective short area burst” is worth seven points toward Speed, while “Speed to turn the corner on outside runs” is worth two points. The components for Blocking varied significantly over time, so I used the RSP’s composite blocking score (adjusted to equalize scores across draft classes). |
---|---|
↑2 | Joseph Addai, Delone Carter, Jamaal Charles, Jonathan Dwyer, Matt Forte, Mark Ingram, Brian Leonard, Marshawn Lynch, Knowshon Moreno, Cedric Peerman, Adrian Peterson, Bilal Powell, Ray Rice, Chris Wells, and DeAngelo Williams. |
↑3 | Reggie Bush, Quinton Ganther, Felix Jones, Maurice Jones-Drew, Jerious Norwood, and Steve Slaton. |